Your Trusted Buyers Agency in Australia
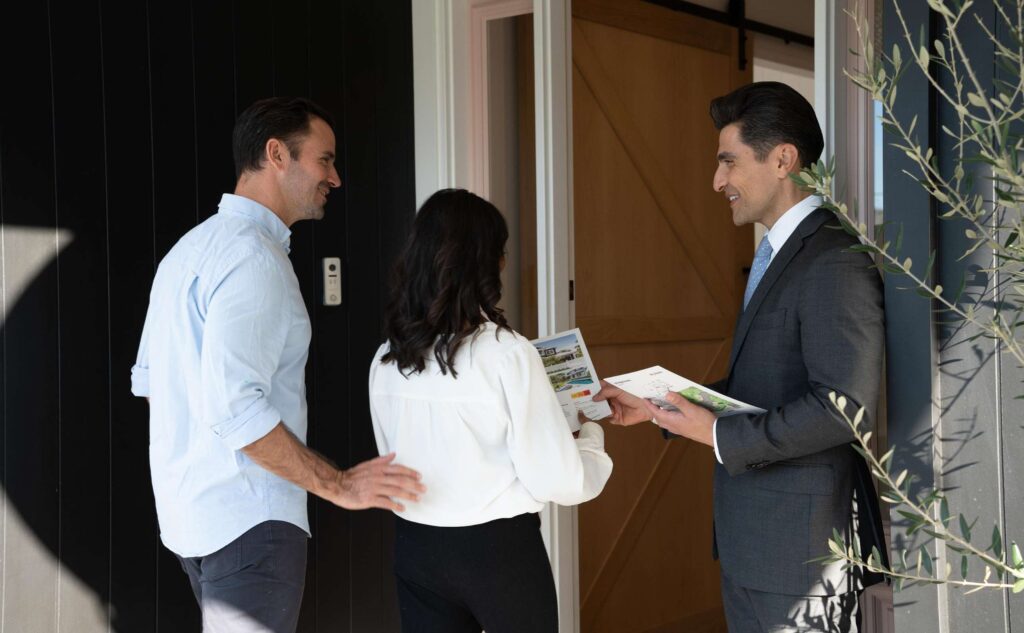
About Us
We pride ourselves on being Australia’s trusted buyers agency, specializing in helping individuals and businesses achieve their property goals. Whether you’re looking for your dream home, an ideal commercial space, or the perfect investment property, we offer expert guidance tailored to your needs.
What Our Clients Say
“Plastica Store made the entire buying process effortless! Their expertise in the Sydney market helped us secure our dream home at a great price. Highly recommend!”
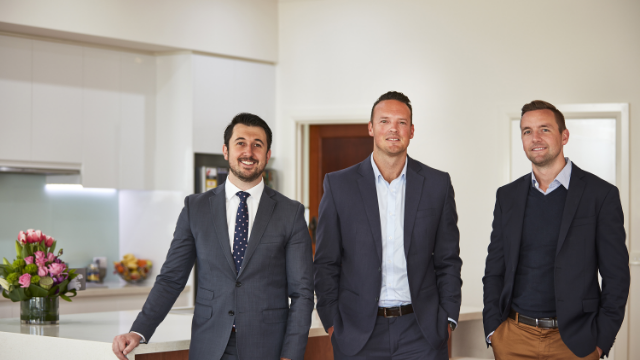
Why Choose Us?
- Expert Local Knowledge – Our agents have unmatched insights into Australia’s property markets.
- Time-Saving Approach – We do the heavy lifting, saving you time and effort.
- Negotiation Expertise – We secure properties at the best possible value.
- Client-First Focus – Your goals and preferences are always our priority.
Our Services
We offer comprehensive buyers agency services tailored to meet your property needs. Whether you’re searching for a home, a commercial space, or an investment property, we provide expert assistance at every step.
01
Residential Property Buyers Agency
We help you find the perfect home, whether it’s your first property or a dream upgrade. Our team handles market research, property inspections, and price negotiations, ensuring a seamless experience.
02
Commercial Buyers Agent
Looking for the ideal space for your business? We specialize in sourcing commercial buyers agent properties that match your goals, saving you time and ensuring value for your investment.
03
Investment Property Services
Maximize your returns with our investment property expertise. We analyze market trends, identify high-growth areas, and secure properties that align with your financial objectives.
Get in Touch
We’re here to help you with all your property buying needs. Whether you’re looking for your dream home, a commercial space, or an investment property, our expert team is ready to assist you.